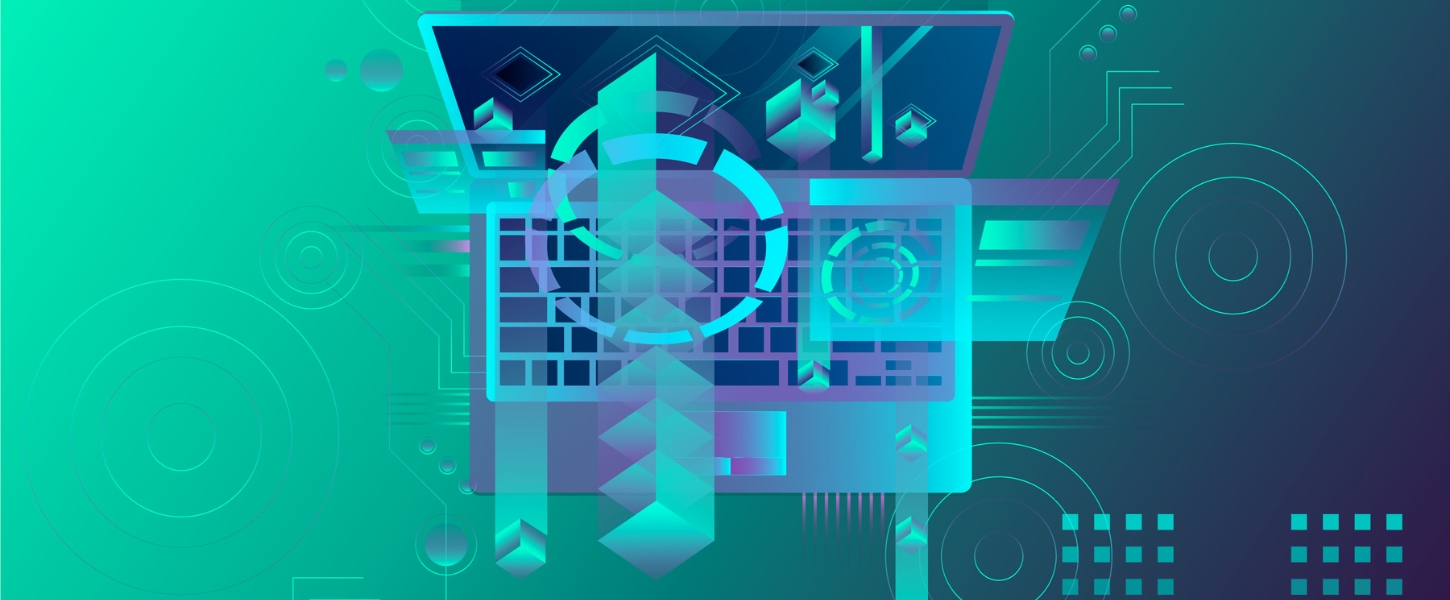
Comparative Testing of RTX 30 GPUs for AI/ML Server Performance
Affordable GPU Servers for AI/ML Computing — Powered by BigCloudNet
In September 2020, NVIDIA introduced the GeForce RTX 30 series based on the Ampere architecture. NVIDIA has taken a nice departure from tradition in pricing this generation, with the aim of facilitating access to AI computing without the builders being socked with the overprice impeding price rise in previous generations. A watershed moment for AI developers who would now get near-Titan RTX performance at rock-bottom prices. With the GeForce RTX 30 series cards, data scientists and machine learning practitioners are able to train bigger and more complex models faster without raising their infrastructure budget.
Fast Deployment of GPU Server from BigCloudNet.
BigCloudNet provides powerful GPU servers with professional Nvidia RTX 5500/5000/A4000 cards installed on them, able to be verified and deployed immediately or custom configured to suit your wishes. Also, we offer VPS hosting with gifted GPU cards — guaranteeing your dedicated GPU is only for you and no one else. GPU performance is stable and powerful in both virtual machines and dedicated servers.
Power in new ways with the series at your fingertips:
- RTX 3090: 10,496 CUDA cores, 328 third-generation Tensor Cores, 24 GB GDDR6X
- RTX 3080: 8,704 CUDA cores, 272 Tensor Cores, 10 GB GDDR6X
Irrespective of the initial shortages in the market, BigCloudNet quickly integrated GPU servers with the greatest GIGABYTE RTX 3090/3080 TURBO into our datacenters to make this cutting-edge technology available anywhere in the Netherlands and Moscow.
Sweet spot for AI computing: RTX 30
The hi-end GeForce RTX 3090 and RTX 3080 are regarded as a massive jump from their ancestors in terms of performance, compared with previous RTX 20 series with Turing architecture. They also perform much better than their former diehard "community favorites," GTX 1080, and GTX 1080 Ti. The ultra-premium cards such as the A100, A40, Titan RTX, T4, and V100 would no doubt offer superior top-end AI power, but the cost becomes exorbitantly out of reach when ranked against all of these. The unconditional value that an RTX 30 series offers is as it closes most of this gap in performance.
Case Study: Face Reenactment and Neural Network Training
We tested our Face Reenactment model (made with U-Net + ResNet + SPADE normalization) on GPU servers equipped with RTX 3090 and RTX 3080, running them through the Titan RTX and GTX 1080.
Key Findings:
- The RTX 3090 was knocked almost right up to the throat with the Titan RTX — all this at a fraction of the cost.
- The RTX 3080 did not quite match up to the 3090, but it readily outran the GTX 1080 Ti.
- The difference in memory size (24GB to 10GB) is critical due to large batch training.
From a worth to value perspective, rental of an RTX 3090 server comes first where AI works are demanding, putting it together as the most affordable price point for developer researchers alike.
Practical Insights: GAN Training & Performance Boosts
In another series of tests involving the training of GAN models through PyTorch:
- The RTX 3090 gets 65% better performance from the RTX 3080 at its best.
- Even then, the RTX 3080 is worlds apart of the GTX 1080 Ti and can be a good trade-off for those reluctant to spend.
The RTX 3080 is still an affordable entry point for prototyping GANs and testing deep learning architectures. But, the RTX 3090 definitely doubles down when it comes to big jobs and heavily loaded production-ready models.
Vision Tasks: Training and Inference Benchmarking
Using modern PyTorch frameworks by NVIDIA, we evaluated RT3090 and RT3080 against the might Tesla V100 (16 GB HBM2):
- Training: The RTX 3090 was only 15%-20% slower than tar for commercial applications with cheap pricing.
- Inference: RTXs delivered fast and reliable performances ready for deployment of trained models.
For this reason, all companies working in computer vision can therefore afford BigCloudNet RTX 30 GPU servers to avail premium results at small costs compared to traditional GPU server rentals.
Detection Training and Model Deployment
The RTX 30 cards were tested for object detection tasks, in particular RetinaNet with ResNet50FPN, and:
- Sluggish performance compared to many costlier choices.
- Greatly increased prototyping and production cycles.
RT 3080s were thus ideal for low-budget rapid prototyping, while RT 3090s were perfect for full-scale deployment of the trained models.
Conclusion
The GeForce RTX 30 series heralds a new age of AI and ML development.
- When memory-intensive workloads such as medical imaging, large-scale GANs, and deep learning models are at stake, the RTX 3090 comes into its own.
- The RTX 3080 is attractively priced for researchers, startups, and businesses developing prototypes or lightweight models.
With respect to the current extremely high prices of HBM memory-based solutions (like the A100s), investing in GPU servers based on RTX 3090 or RTX 3080 inks through BigCloudNet ensures maximum returns are assured for at least the next few years.
When upping AI capabilities or starting from scratch, BigCloudNet's GPU server solutions will give you the performance edge required—all at pricing suddenly within reach.