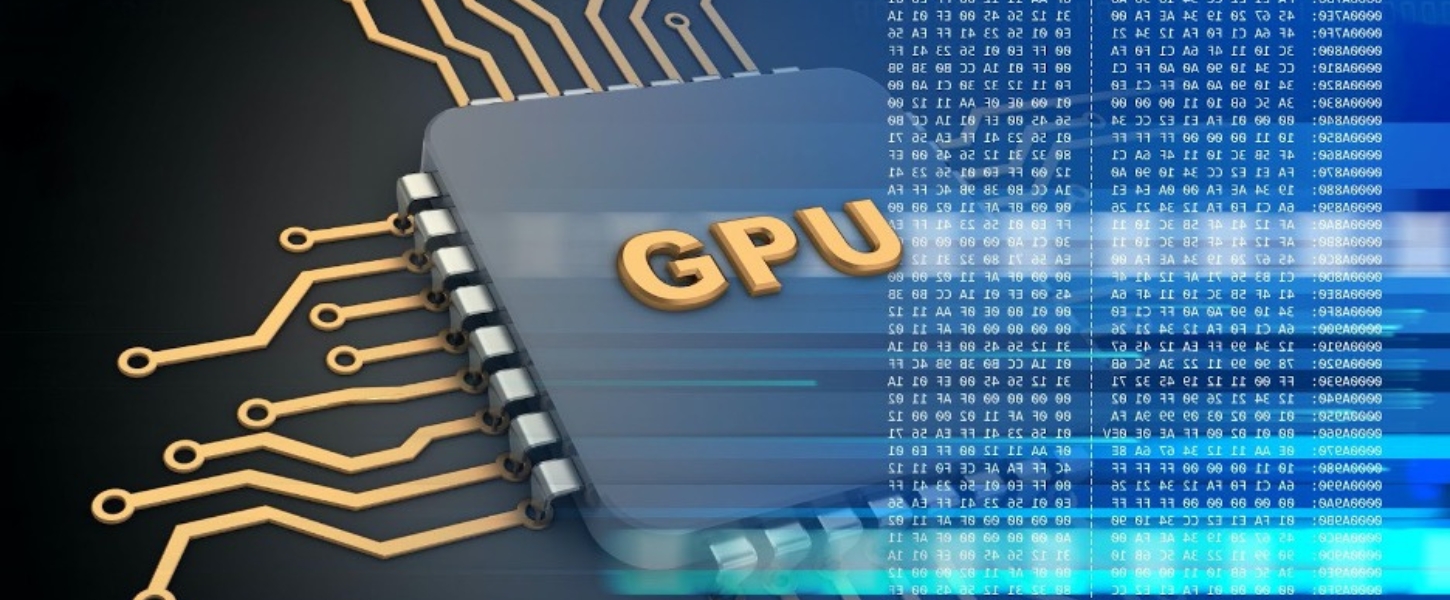
AI-Optimized GPU Server Configurations for Every Task
GPU versus CPU—the Key Differences
Starting from very basics—the Central Processing Unit (CPU) is the computer's brain that executes calculations ranging from very complex calculations to controlling inputs and outputs from all devices connected to the computer. The CPU is designed to perform detailed logical operations, run very complex codes, and carry out mathematical calculations. Over the centuries, these CPUs have been improved, gaining power and memory; however, the basic structure has remained unchanged, making them inefficient for processing tasks that require large-scale parallel processing.
This is where GPU comes into play, which is often very well referred to as competing with our eyes in vision processing for the computer. It is basically made to render graphics. While CPU might be in charge of processing a few complex tasks, GPU is built to take upon itself to accomplish a great number of simple concurrent tasks. Such computational capability is extremely useful in applications in demand, such as 3D rendering and the video graphics. The most important thing is that the parallel computing capabilities of the GPU extend way past graphics, making them also very worthy of AI and ML tasks where huge amounts of data have to be processed at the same time.
Advantages GPUs Have Over CPUs
The general-function CPU might be deemed incapable of carrying some tasks, but when large-scale data analysis, parallel computing, and real-time processing are concerned, the GPU becomes a true powerhouse. Due to the parallel computing capabilities of the GPU[when used with CPU], the execution time is significantly shrunk, since the GPU can execute many processes in parallel, whereas a CPU does so sequentially. Thus, in a hybrid computing system, the use of CPU and GPU cuts down processing time greatly and assigns lighter jobs to the GPU to enable the CPU to concentrate on heavier work.
GPU Role in AI Processing
The emergence of AI has meant an increased dependence on GPU-based processing. While CPUs exploit a small number of powerful cores, GPUs utilize thousands of cores for executing simple tasks in parallel, which is extremely useful for ML and DL requiring the processing of vast amounts of data and detection of patterns.
Clearly, Deep Learning entails sets of complex algorithms that analyze very large datasets to recognize and classify novel input. Such novel input is widely utilized in speech recognition, driverless vehicle applications, and medical diagnostics. The GPU computing process, being parallel, therefore accelerates the training of these algorithms, making the training quite fast and cost-efficient compared to a sole reliance on the CPU way of training.
Whereas a CPU may be found struggling to meet a task that requires the simultaneous processing of millions of data points, this is where GPU outperforms. Looking at the extreme contrast of the rolling power of modern GPUs, including NVIDIA's latest quotations with 3,500 cores, and the luxury of high-end CPUs offered by Intel with only about 30 cores presenting a clear-cut GPU edge over AI workloads.
AI-Enhanced GPU Processing
AI-assisted GPU computing provides new opportunities for businesses. AI and ML mean better data-driven choices for all kinds of decisions—from price adjustments based on trends to automation of customer service. Businesses make use of the user-friendliness and speed with which GPUs can handle huge volumes of data.
Some applications of AI-enhanced systems that cut down time and costs include Predictive Modeling, Forecasting, and Data Entry Automation. The best thing about implementing AI is that businesses can set it up without investing too heavily in building an entire AI infrastructure.
Should You Go Ahead and Switch to GPU?
Today, in the data-rich world, companies are drowning in information. AI, ML, Deep Learning tools are some invaluable assets to get actionable insights from these humongous datasets. These tools help businesses to stay agile, keep pace with rapid changes in the marked environmental conditions, make informed decisions, and facilitate the smooth functioning of business operations.
When Should You Shift to GPU?
The decision to shift to GPU-based servers more so depends on the tasks at hand with contending complexity and scale. If you are working within the remit of AI or Deep Learning tasks, utilizing GPU would go a long way toward rendering efficiency. Now, let us take a glance at some tailored GPU Server Configurations meant to satisfy different requirements.
GPU Server Configuration Customization
Every AI task pairs with its computing environment. From entry-level experimentations to enterprise solutions for AI, here's how you can customize your GPU server configuration:
Starting with AI? A Beginner-Friendly Setup
For those coming into AI, a Tower GPU Server with up to five consumer-grade GPUs should be more than adequate to get you started. This configuration is quite sufficient for testing and experimenting with AI and Deep Learning without much upfront capital investment. It can be scaled onward as your AI needs grow, so you can add storage, memory, and additional GPUs as required.
Ready for the Big Leagues? A Commercial-Grade Upgrade
In the latter stages of development before the public launch, an environment fulfills the need of an upgrade to something stronger. The most reliable high-performance servers are marketed with commercial-grade GPUs like the NVIDIA Tesla™. Such configurations are built for mission-critical applications, enabling continuous, trouble-free operations of AI models, thus providing trouble-free experiences for customers.
Need to Crunch Big Data? Go Fast, Go Efficient
Consider a single root CPU server with four GPUs for large AI workloads like Neural Networks and NVIDIA GPUDirect™ RDMA Applications. With this setup, maximum processing power is combed with efficiency. In high data throughput applications, pairing a good CPU such as Intel® Xeon® Scalable processors will give you performance and scalability.
Handling Massive Datasets? Scale-Up!
If your business demands high computing and huge data storage, the ideal configuration should be dual-root architecture with 12 hot-swap drives and dual-Xeon CPUs. This architecture fits perfectly in rapidly processing large data sets in AI and deep learning applications. The two CPUs paired with enough storage capacity guarantee a high-speed and reliable process when dealing with huge amounts of data.
Do you need raw power for deploying an AI in production?
When needing an AI for production purposes, including but not limited to Deep Learning and full-scale model training, a server must support up to ten GPUs. Quite a facility in a 4U rack mount chassis can provide the high throughput required for cutting-edge AI research and development. Yet, GPUs will connect via PCIe switches, optimized for low-latency, high-bandwidth operations using technologies such as GPUDirect RDMA for maximum performance.
Big Cloud Net: Scalable GPU Servers to Your Service
Are you looking for a highly scalable and efficient GPU circuit for your AI and ML work? Big Cloud Net provides scalable, robust GPU solutions for computing requirements in various AI and ML projects. Whether you are an aspiring entrepreneur for AI or want GPU mining for an industrial-scale big firm, Big Cloud Net has GPUs on-demand from anywhere to anywhere. Transform AI capabilities with optimized configurations for fast data processing and enhanced AI/ML performance - right from proof-of-concept to fully operational deployment, Big Cloud Net can charge your application with the requisite power and flexibility.